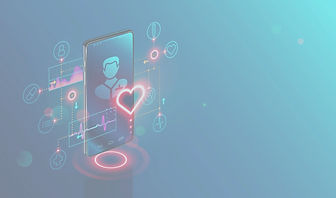
AIHEALTHCARE
Exploring the Impacts and Implications of Artificial Intelligence & Medical Technology
Ryan Nguyen, Pooja Thorali, Melanie Kuo, Kassidy Gardner
![]() |
---|
AI IN HEALTHCARE
We have seen the rise of AI models in recent years, with new technology such as chatGPT-3 receiving more and more attention in both the research and public space. With this growing interest, we have also seen the implementation of AI in several fields, one important field being healthcare. As such, there is a need to examine the potential biases and ethical concerns of such implementation.
How can AI in Healthcare be biased?
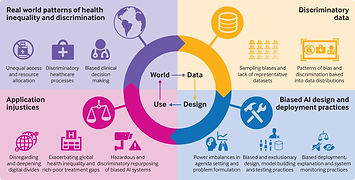
AI has been known to be biased as a result of the training data used to create the model. Several examples of this can be seen from the recent release of chatGPT-3, where asking the model to write a joke concerning marginalized groups, the results were often discriminatory or offensive. But how does this bias apply to healthcare?
​
One example of this is how radiologists experimented with AI algorithms to help diagnose patients using chest radiographs. What they found was that Black and Hispanic women were reported to be underdiagnosed in CXR scans. (Seyyed-Kalantari, et al.) The algorithm giving inaccurate diagnoses can cause certain communities to receive less treatment than needed, leading to inequality in how different groups are treated in healthcare.
"What they found was that Black and Hispanic women were reported to be underdiagnosed in CXR scans"
(Seyyed-Kalantari, et al.)
AI has the potential to massively change the healthcare industry, but with it comes many challenges. DeCamp and Lindvall argue that there are three main challenges, which are:
​
-
The fact that an adaptive AI algorithm, which learns and improves in performance over time, can become biased if it learns from disparities in care or uncorrected biases in the broader healthcare system. This means that an algorithm developed to operate fairly in one context could start producing biased results in a different context, leading to further worsening biases in prediction.
-
How AI interacts with clinical environments that include their own implicit and explicit biases. For example, even a perfectly fair algorithm can perform unfairly if it is only implemented in certain settings, such as clinics serving mainly wealthy or white patients. This is due to the phenomenon of privilege bias, which disproportionately benefits individuals who already experience privilege of one sort or another. Additionally, the phenomenon of automation bias can lead to busy clinicians following AI-based predictions unquestioningly, leading to biased outputs.
-
How AI models choose what is intended to promote, which is the outcome or goal of interest. If the outcomes or problems chosen to be solved by AI do not reflect the interests of individual patients or the community, this is effectively a bias. The authors suggest that it is important to carefully consider the goals of the AI model to ensure that they reflect the interests of all patients and the community as a whole.
​
In conclusion, the authors argue that even seemingly fair AI predictive models implemented within electronic health records can involve latent biases, which can affect their accuracy and effectiveness. They suggest that it is important to address these latent biases before the widespread implementation of AI algorithms in clinical practice to ensure that AI can be used safely and effectively in healthcare.
​
Source: https://www.ncbi.nlm.nih.gov/pmc/articles/PMC7727353/
Challenges in implementing AI in Healthcare
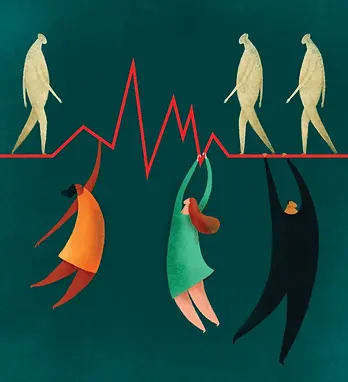