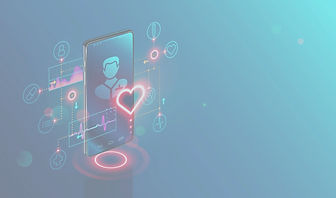
AIHEALTHCARE
Exploring the Impacts and Implications of Artificial Intelligence & Medical Technology
Ryan Nguyen, Pooja Thorali, Melanie Kuo, Kassidy Gardner
Underdiagnosis bias of algorithmic chest radiographs
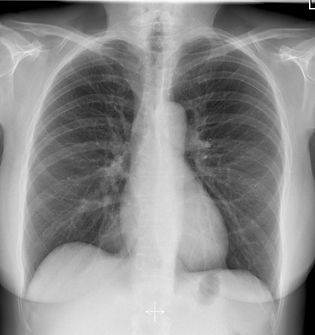
Study/Technology Details
Underdiagnosis is defined as a condition that is diagnosed less often than it actually occurs, meaning that these populations were disproportionately deemed healthy when they should have been flagged to receive clinical treatment. In a study by Seyyed-Kalantari et al. focusing on biases and underdiagnosis problems in chest X-ray prediction models, the researchers discovered that patients who belonged to more than one subgroup population experienced compounded biases and underdiagnoses from these recommendations.
​
Affected Groups
The groups that experienced the highest rates of underdiagnosis from these algorithms were:
-
Patients under the age of 20 with Medicaid insurance
-
Black patients who are under the age of 20
-
Female patients who are 20 years and younger
The study also highlights the importance of recognizing that the data is representative of biases that exist in our society and healthcare systems, so models and algorithms trained on this data will perpetuate these biases.
​
Study/Technology Details
The article discusses a study on the potential for sex and racial bias in artificial intelligence (AI)-based cine CMR segmentation, which is used for functional quantification. The study used a deep learning (DL) model to automatically segment ventricles (the heart pumps) and myocardium (heart muscular tissue) from cine short-axis CMR images of 5,903 subjects from the UK Biobank database. A Cardiovascular MRI can take non-invasive images of a person's inner cardiovascular system structure and functionality.
​
Affected Groups
Results showed that the AI model was biased against minority racial groups, even after correction for possible confounders, and that race was the main factor that explained the overall difference in Dice scores between racial groups. The study highlights the importance of fair AI in medical imaging and the potential for bias in AI models trained on imbalanced databases.
​
Source: https://www.ncbi.nlm.nih.gov/pmc/articles/PMC9021445/
Inequalities in Cardiac Magnetic Resonance Imaging Deep Learning Segmentation
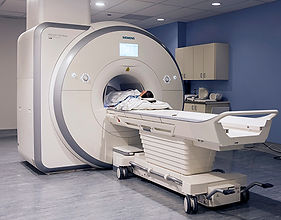
Racial Bias Found in a Major Health Care Risk Algorithm
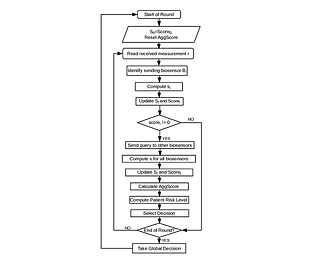
Study/Technology Details
A technology that is being tested and possibly integrated into healthcare is a healthcare risk-prediction algorithm, where the algorithm determines which patients needed higher amounts of care and which patients were at a lower risk for health problems allowing hospitals to have effective care management.
​
Affected Groups
This healthcare risk prediction algorithm was used on more than 200 million people in the U.S. and demonstrated racial bias because it relied on a faulty metric for determining need. The algorithm helps hospitals and insurance companies identify which patients will benefit from “high-risk care management” programs, but researchers found that black patients tended to receive lower risk scores even when they spent the same amount as white patients. The study highlights the need for AI developers and users to be aware of possible biases in data and programmed assumptions and to conduct basic audits before their product touches a real patient.
​
Source: https://www.scientificamerican.com/article/racial-bias-found-in-a-major-health-care-risk-algorithm/
Study/Technology Details
This study focuses on the potential benefits and drawbacks of implementing “biological (sex) and socio-cultural (gender) aspects” into machine learning healthcare prediction models, (Cirillo et al., 2020). Sex and gender impact many different aspects of health such as risk factors, disease prevalence, symptoms and manifestation, prognosis, efficacy of treatment, and more. This would be very useful to integrate into prediction machine learning models to increase the accuracy of results as well as the usefulness in clinical decision making.
​
Affected Groups
With that being said, there is a potential for these systems to reinforce biases that exist both in the real world and in the data and data collection. According to this study, early biomedical research often excluded women and focused on male subjects, creating a lack of research that is relevant and useful to women. This lack of representation in studies has continued, such as a digital biomarker test for Parkinson’s in which only 18.6% of the subjects were women, which would therefore affect any sort of prediction algorithms that were trained on this data. The researchers of this study urge for the need for explicable justification to explain how the AI models come up with a certain output so that biases in sex and gender differences can be better identified.
​
Sex and Gender in machine learning healthcare prediction models
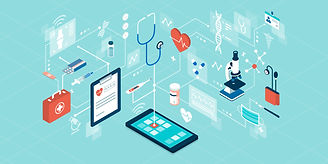